Introduction
Did you know that 75%of gen AI users are looking to automate tasks at work and use it extensively for communications? AI is no longer a new technology, several organizations across industries have been using it for over a decade to optimize operations and provide an engaging experience for customers.
Over the last three years, many companies have actively embraced gen AI, AI agents, and chatbots to amplify their services and provide a holistic experience for customers through their AI-powered services. Aspiring data science professionals have had the opportunity to learn gen AI to boost their career prospects and land better jobs. They have been highly successful in understanding the complexities of large language and deep learning models. This has helped them scale up in their career and become nearly indispensable in the present market which is highly competitive. If you are looking to learn more about gen AI, here is a deep dive that delves into the core principle of gen AI, its architecture, functionality, and applications.
-   ● Basics of Gen AI
-   ● What is a Generative Model?
-   ● Architecture of Gen AI
-   ● Generative Adversarial Networks
-   ● Autoencoders
-   ● Variational Autoencoders
-   ● Recurrent Neural Networks (RNNs) and Long Short-Term Memory
-   ● Applications of Generative AI and Future Trends
Basics of Gen AI
Nearly 70% of non-AI users are ready to use gen AI if they are more well-versed with the technology. This means that if you are well versed in gen AI technology, you can educate them and make sure that they harness the power of this technology to help them achieve their business goals with ease. Generative AI uses deep learning methods to better understand vast datasets and patterns and provide an appropriate response. Unlike traditional AI that needs to be programmed, gen AI has the unique ability to learn from data, make predictions, and generate new content. At its core, it is geared toward neural networks which are the building blocks of deep learning.
Gen AI is a field within AI that specializes in creating systems that are capable of creating new content like text, images, videos, audio and more. They achieve this by learning the underlying data patterns and structures that they are trained on. This empowers these systems to create original content that is tailored to each user’s input.
What is a Generative Model?
A generative model generates inputs based on the trained data. There are a plethora of different models, each with a unique approach to learning and creating new data. Variational Autoencoders (VAEs), autoregressive models, and Generative Adversarial Networks (GANs) are some of the most commonly used generative models. With industries on the lookout for candidates with unique skills in data science and generative AI, aspiring gen AI professionals are sure to find an appropriate job that pays well. This technology helps businesses generate a lot of revenue, so, many companies pay a certain percentage of the revenue as an incentive to their employees. There will surely be a boom in gen AI jobs as 67% of IT leaders are looking to implement gen AI in their business within the next two years.
Architecture of Gen AI
The core principle of gen AI is to learn about its architectures which will give you a clearer picture of how the AI model functions. Most importantly, you can understand what makes these systems generate high-quality inputs consistently with improved accuracy and attention to detail. The core architecture of gen AI comprises application layer, data platform layer, orchestration layer, model layer and hub, and infrastructure layer.
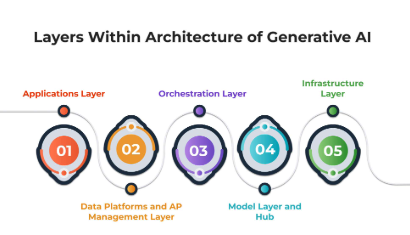
Generative Adversarial Networks
There are two neural networks, a discriminator, and a generator in a GAN. These layers are trained simultaneously. The generator is trained to generate output that is indistinguishable from real data and the discriminator is trained to differentiate between real and fake data. This adversarial training process empowers GANs to generate realistic data with ease.
The generator takes latent vector or random noise as input and generates data samples accordingly. It learns to generate data that is similar to the real data from the training set. The discriminator is trained on a combination of real and fake data sets, enabling it to assign high probabilities to real samples and low probabilities to fake ones.
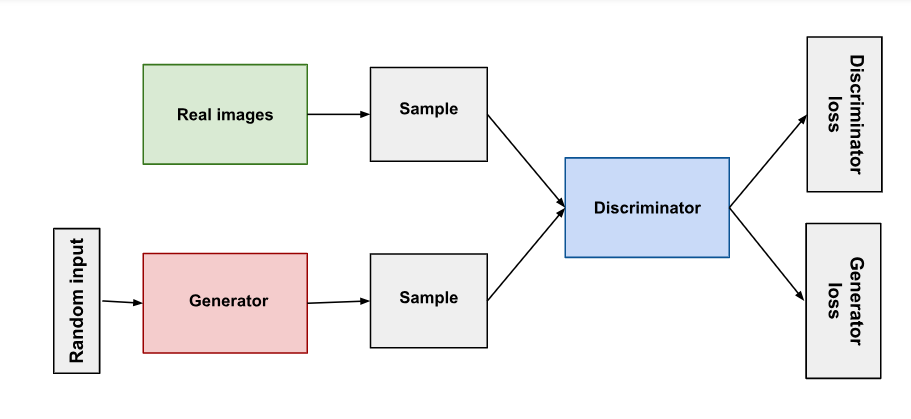
In adversarial training, the discriminator and generator are trained simultaneously in a minmax framework. The generator tries to produce data samples that are indistinguishable from real samples. This helps in maximizing the accuracy of outputs. Training GANs can be quite challenging as there could be issues where the generator produces limited samples and vanishing gradients. Gen AI experts use deep convolutional GANs-DCGANs, different architectures, regularization methods, and alternative loss functions. GANs are widely used in various tasks like image generation, image-to-image translation, style transfer, super-resolution, and more.
Autoencoders
In unsupervised learning, autoencoders have played a pivotal role in compressing input data by compressing input data and reconstructing it. This is a unique feature that has been used in image denoising and feature extraction. Autoencoders and neural network architectures are widely used for efficient data representations. They compress input data into a lower-dimensional latent space and reconstruct the original input data from this representation. An encoder and decoder are the two major components of an autoencoder.
Autoencoders are neural network architectures that understand compact data representations without the need for labelled training examples. They are applied in various domains such as computer vision, natural language processing, and signal processing.
Variational Autoencoders
Variational Autoencoders (VAEs) is a generative model that learns compressed representations of input data or latent space. This space could be used to generate new data samples resembling the training examples. VAEs are highly useful in generating images and are widely used in various creative applications. They are used in generating artwork and creating realistic faces. They offer a powerful representation for learning rich data representation of complex data distribution.
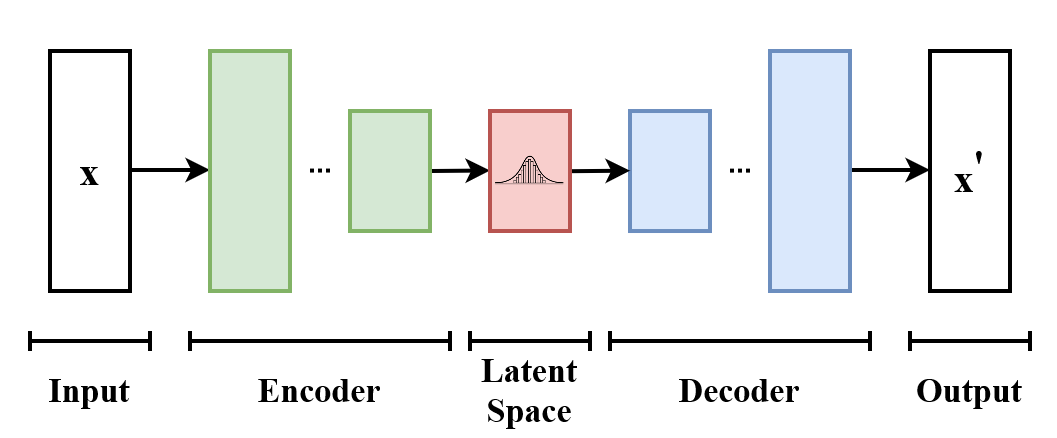
Recurrent Neural Networks and Long Short-Term Memory
Recurrent Neural Networks (RNNs) are a class of neural network architectures designed to handle sequential data by maintaining an internal state or memory. Unlike traditional feedforward neural networks that process each input independently, RNNs capture temporal dependencies within sequences. This makes them best suited for time series prediction, natural language processing, and speech recognition. There are many variations and extensions in RNNs, which include LTSM (Long short-term memory), and gated recurrent units that address some limitations of basic RNNs.LTSMs are a type of RNN architecture designed to address vanishing gradient problems and capture long-term dependencies in sequential data.
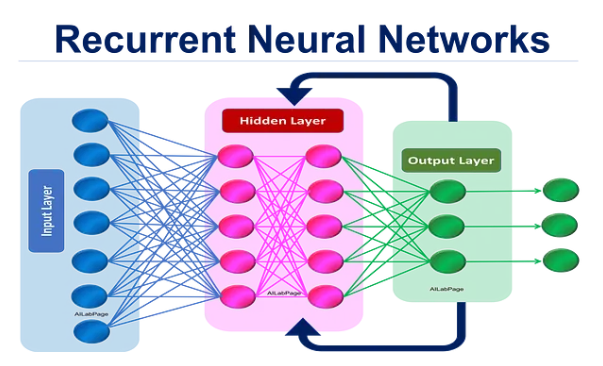
Applications of Generative AI and Future Trends
Generative AI has been widely used to create amazing artwork, design unique logos, compose music, and create realistic virtual worlds. Artists and designers are able to augment their creative processes with AI by pushing the boundaries of their imagination. However, as AI systems become more advanced, they generate fake content and use generative models to maintain data integrity and trust in the digital realm. There are several new trends in gen AI as they are likely to witness new trends that may include advancements in generative models, improved techniques for fine-tuning the generated output and creating more accessible tools for artists and developers.
If you are looking for a hand-on approach to learn generative AI, you can take up the Generative AI course by Eduinx, a prominent edutech institute. Eduinx provides a practical approach to learning generative AI through capstone projects. Their mentors are non-academicians with over a decade of experience in AI will help you understand complex concepts with ease. You can become industry-ready and equipped with the right skills to help you stand out from the competition. Reach out to Eduinx for more information on the course.
Reference links:
https://medium.com/@theagipodcast/unraveling-the-magic-a-deep-dive-into-generative-ai-0e34cbf89895 https://dasha.ai/en-us/blog/what-is-generative-ai-a-deep-dive-into-its-mechanisms